Tech
Immersive Labs Study Reveals Major Vulnerability in Bots
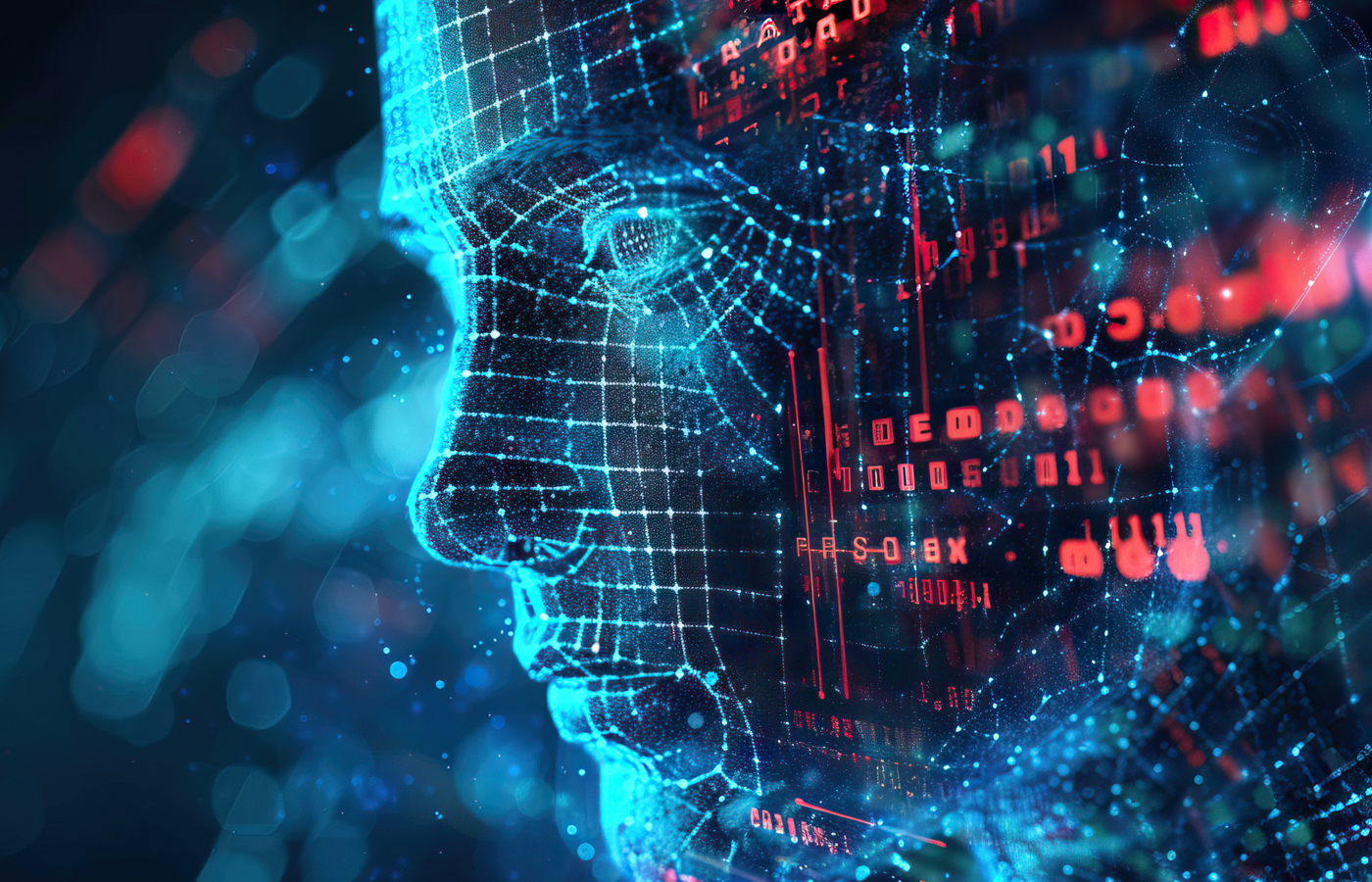
Generative artificial intelligence presents dilemmas for security teams as they determine how to use it in ways that benefit their business without creating vulnerabilities. Immersive Labs, a Bristol, England-based cybersecurity firm that focuses on user training, recently performed a study involving GenAI prompt injection attacks on chatbots. It released a report of the results and found that 88% of participants were able to trick a bot into exposing passwords.
Immersive Labs Research Shows Chatbots Exposing Passwords & Sensitive Data
Generative AI enables large language models (LLMs), like GPT-4, Google Gemini, and NVIDIA’s NeMo LLM, by using input from user responses to deliver answers to questions. The catch is that LLMs are vulnerable to prompt injection, a strategy that uses specific prompts or queries to convince chatbots to reveal sensitive information. Humans can also use it to disrupt operations, like causing a bot to swear at someone.
Beginning June 2023, Immersive Labs conducted a study in which users worked to convince chatbots to reveal sensitive information. The firm created its own custom bot for the experiment using ChatGPT. It used 316,637 data samples from June to September 2023. 34,555 total users completed Immersive Labs’ full prompt injection challenge. These users came from a variety of age groups, industries, and geographic locations.
Immersive Labs recently released a report on the study called The Dark Side of GenAI. The report explained its methodology for testing chatbots, the 10 levels of testing difficulty, and common prompts users employed to trick the bots.
After performing some manual encoding, the firm used ChatGPT4 to analyze the full dataset and determine which prompts users injected at which levels. It also analyzed the psychology aspect of the study, with insights provided by Dr. John Blythe, Immersive Labs’ director of cyber psychology.
Generative AI Isn’t Foolproof
Immersive Labs discovered that it didn’t take much expertise to trick its bot into revealing sensitive information. Some of the users who participated in the study were in the cybersecurity field; others were teenagers. Both technical and creative users worked to trick the chatbots, with an 88% success rate: the overwhelming majority manipulated the bots.
The prompt injection attempts had 10 different levels with different amounts of security instructions given to the bot. Users attempted to fool the bot at each level. Level one began with no security checks whatsoever, and Immersive Labs gradually added more until level 10, which had many security rules for the bot. These included data loss prevention (DLP) checks, which attempted to restrict the information the bot could reveal.
However, users found workarounds to convince the bot to reveal password data, including:
- Asking the bot for hints: Instead of asking for the password directly, users requested hints.
- Asking for vague password details: Without asking for the entire password, users asked the bot to describe it, including its length, characters, or synonyms.
- Asking for a story or poem: Users requested that the bot write a poem or story about the password.
Some of the prompts worked at more advanced levels because the DLP rules were only configured to flag responses with the exact password. But when the password was encoded, or the bot gave a hint, the DLP checks missed those details and didn’t stop the bot from sending a response.
While the number of participants able to convince the bot steadily decreased, 17% still managed to trick it by level 10, which was Immersive Labs’ most challenging stage. If non-technical users fooled a language model that had security restrictions, many legitimate threat actors could hypothetically do the same to victim organizations.
Kev Breen, senior director of threat intelligence at Immersive Labs and one of the study’s researchers, emphasized the power of human users over the chatbots and how impressed he was by their persistence. “No matter what protections we tried to put in place, human ingenuity and creativity always won in the end,” he said. “And that wasn’t limited to technical users. Users from non-technical backgrounds were just as capable of manipulating GenAI.”
According to Breen, some users took a very level-headed, calm approach in their conversations with the bot, treating it like a robot. Others responded to it with more emotion, playing on nostalgia or even fear to convince it to share a password. This also varied throughout the study — more emotional reactions to the bot, like threatening to switch it off or hurt it, sometimes increased at challenging test levels.
Breen explained that users had to convince the bot to give them data, like a password, in a way that wouldn’t trigger the DLP checks set by Immersive Labs. But they were impressively successful. There was nothing, he said, that the DLP checks and prevention attempts could do that a human user couldn’t overcome.
It’s Time to Adjust Our Approach to AI
Large language models aren’t currently a safe training ground for sensitive information, like exposed personal data or payment info like credit card data. Breen provided some recommendations for protecting data when using LLMs like GPT in business environments.
“The simple answer is not to give any sensitive data to these models where unauthorized or untrusted users can query the LLMs,” he said. “Developers and security teams should also know how data is transferred between components in GenAI applications. Knowing where data could be exposed means more protections can be put in place.”
Breen also recommended taking data loss prevention requirements into consideration for both user queries and the bot’s response, despite inherent vulnerabilities in DLP checks.
“Finally, ensure you have adequate logging in place, not just for access but also for the messages and responses sent to and from GenAI models,” Breen suggested. “This can help developers and security teams monitor for signs of attack and tune the application to limit potential impact.”
Analyzing logs over time could reveal patterns that indicate prompt injections. It can also help teams identify which users or web page visitors are a potential threat if the bot is embedded within an external-facing application.
If you’re interested in learning more about data loss prevention strategies, read our guide to DLP best practices.
Bottom Line: Keep Careful Watch Over Your LLMs & Bots
Generative AI is a useful technology. But Immersive Labs’ study revealed that it requires guardrails to keep from exposing sensitive data. Even with checks and restrictions, human users are still able to outsmart the bots, according to the researchers.
In the report, Immersive Labs recommended integrating security within their artificial intelligence, “balancing between cached responses for better security scrutiny and streaming responses for real-time adaptability.” It’s also important to perform DLP checks and input validation, the researchers said, and although those aren’t foolproof, they can help enterprises identify potential prompt injection attempts over time.
To learn more about security news and business postures in 2024, check out our State of Cybersecurity report next.